Ideal Unified Namespace Pilots

Two ideal UNS pilots are Continuous Process Verification (CPV) and Machine Learning. CPV because it involves batch to batch comparisons that may have been manufactured in different ways or locations. Customers ask, “How can I measure the quality of my product if the critical process parameters are measured differently?” Machine Learning is a great UNS example because you’re bringing in data from multiple systems and UNS is a good way to feed those models.
Machine Learning has demonstrated the potential to extract insights and value from data; however, relative to other domains, manufacturing has been slow to realize this value. While pilot studies and proof of concept projects abound, scaling these solutions has been challenging. When asked, manufacturers cite inadequate data quality, poor data integration, and weak data governance as major reasons for the inability to easily scale solutions. Specifically, poor data integration and weak data governance are barriers that must be overcome to scale the integration of the multiple OT and IT systems required for deployment of Machine Learning solutions.
The concept of a Unified Name Space (UNS) directly addresses these barriers. UNS draws on modern distributed technology & architecture and combines it with hierarchical data naming and organization. The result is a centralized and standard presentation of OT data to enterprise systems. UNS is a scalable approach that addresses data integration and governance issues, removing two of the main impediments to the wide deployment of Machine Learning models.
While UNS can enable wider deployment of Machine Learning in manufacturing, its value extends far beyond any single use case. Having a single source of truth for manufacturing data can reduce the cost, simplify the deployment, and improve the robustness of any analytics or reporting solution that depends on manufacturing data. UNS should be considered as a key component of any digital transformation strategy.
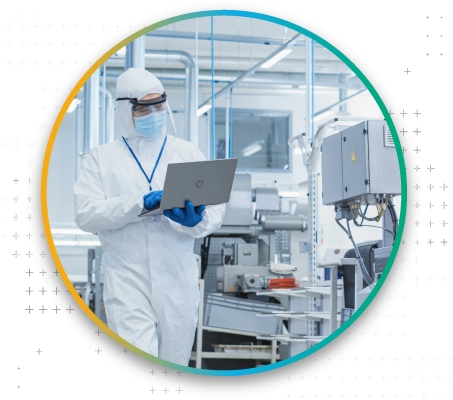